Dr Gareth James: Using Appreciative Inquiry in housing research
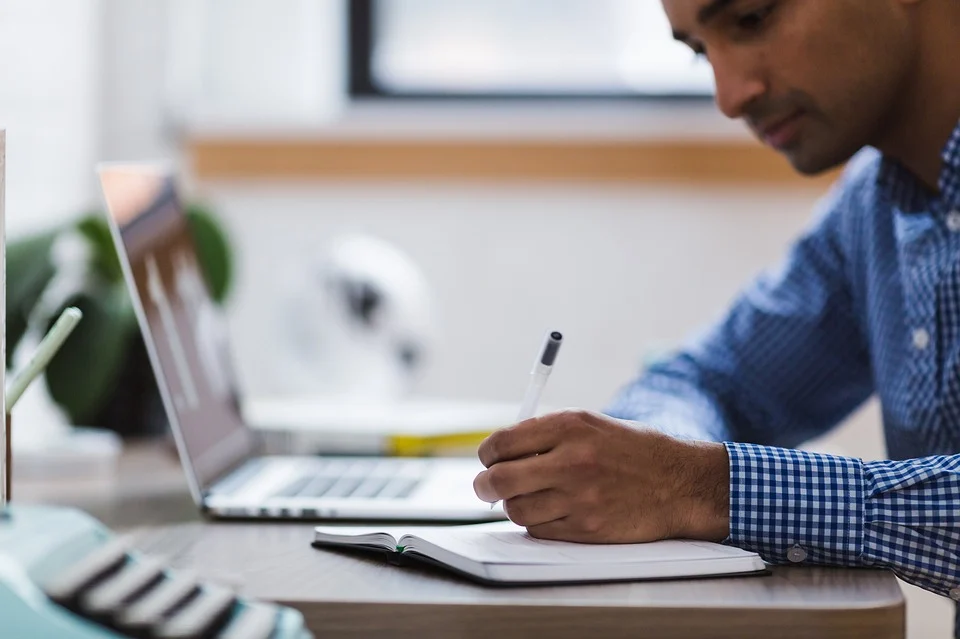
Researchers in the social sciences are increasingly using innovative methodologies to promote collaboration and generate impact. Appreciative Inquiry (AI – not to be confused with Artificial Intelligence) is one such approach. This blog by Dr Gareth James highlights the principles, strengths and limitations of an AI approach, and offers some critical reflections on its use in housing research.
The Appreciative Inquiry (AI) approach
AI has been used across social science disciplines such as social work, community development, and organisational studies. Its adoption reflects a shift from “problem-driven” or “deficit-oriented” research (i.e. research into “barriers” to development or “failures” in policy implementation) toward what social work theorists might call “strength-based” methodologies (i.e. methodologies that aim to leverage existing strengths, within a system for example, with the aim of building on what works). As a methodology, it is similar to asset-based community development. In other words, AI focuses on identifying and amplifying what works well, inspiring participants to envision and co-create a better future.
The collaborative ethos underpinning the AI approach makes it an appropriate choice for co-producing research and policy recommendations with stakeholders. It is both a philosophy and a process, grounded in principles that emphasise collaboration, positive inquiry, and the co-creation of knowledge.
The Five-D Cycle is central to the AI approach. It is a structured framework that guides participants through the inquiry process as follows:
- Define: Clarify the focus and purpose of the research.
- Discover: Explore what is working well by uncovering strengths and successes.
- Dream: Envision an ideal future based on the collective strengths identified.
- Design: Develop strategies and structures to realise the envisioned future.
- Deliver: Implement changes and ensure their sustainability.
This approach encourages participants to reflect on their own knowledge and experiences and to co-design solutions, fostering empowerment and commitment.
Applying the AI approach to housing research
Earlier this year, Madhu Satsangi, Victoria Lawson and I adapted this framework and applied it in our research on land for housing supply in rural Scotland. The project report will be published by the Scottish Land Commission soon.
We used AI with stakeholders to co-produce a set of practical recommendations that would help deliver a 10-year supply of developable land to meet housing need and demand in rural Scotland.
The workshop involved key stakeholders, including policymakers, rural housing enablers, SMEs, and community representatives. The process was structured around the Five-D Cycle, beginning with a questionnaire distributed prior to the workshop.
The questionnaire invited participants to identify barriers to land supply, thus clarifying the focus and purpose of the research. Acknowledging the barriers early in the process allowed us to park them and move forward to positive solutions-focused discussions using AI. This step was crucial for securing buy-in and setting a realistic foundation for the discussions.
The workshop subsequently unfolded in four rounds of group discussions, guided by the Five Ds, resulting in several key insights which helped to inform the research and our recommendations.
- In Round 1 (Discover), participants shared stories of what works well, highlighting strengths in the system, such as strong partnerships, supportive policies, and pragmatic approaches to development.
- In Round 2 (Dream), stakeholders envisioned an ideal future, proposing ideas like creating databases of good practices, adopting proactive land management strategies, and integrating environmental and social governance into land markets.
- In Round 3 (Design), groups identified actionable steps, including revisiting compulsory purchase orders (CPOs) and enabling plan-led land supply.
- In Round 4 (Deliver), participants prioritised immediate actions such as guidance on CPOs, support for SMEs, modular housing development, and knowledge exchange with countries like Canada or Scandinavia.
To stimulate deeper discussion, provocations were introduced in Phase 3. These included proposals for land and tax reforms, such as compulsory sales orders, changes to capital gains tax, and the establishment of a national housing land agency.
Stakeholder feedback
Stakeholders provided positive feedback on the AI process, emphasising its inclusivity and productivity. Participants also valued the opportunity to engage in solutions-oriented discussions and appreciated the structured yet flexible format of the workshop.
For example, in response to our feedback survey, one participant wrote:
As a sector, I often wonder if people just prefer to talk about the problems instead of actually expending energy to actually come up with practical solutions that would actually see the light of day. It was really refreshing to not experience that, and [I] think it was the first time that the positivity and practicality came about because of the chosen process, rather than through constant steering or reminders.
However, participants also expressed caution, noting in particular that sustaining momentum and translating ideas into action remains challenging. For example:
I’m not sure it [the AI approach] quite solves the problem of making recommendations and solutions fully practical. I think they [the outputs from the workshop] are generally much more useable than outputs from other workshops, but [they] will still need work to get to something which actually fits in the ‘real life’ context. But I suspect that the outputs from this workshop are likely of higher and more precise quality.
Some critical reflections on the use of AI in (housing) research
AI offers a structured approach for engaging stakeholders in research, particularly when addressing issues such as housing supply. Its strengths – including collaborative engagement, a positive focus, flexibility, and the ability to generate innovative solutions – make it an effective method for facilitating discussion and developing practical policy recommendations. However, its application requires careful consideration to avoid downplaying barriers or overlooking critical challenges.
As with any qualitative method, the outcomes of AI depend significantly on the participants involved. Questions regarding the representativeness of stakeholders, the potential for bias, and the risk of dominance by specific interests are particularly pertinent. It is crucial, therefore, to ensure that quieter or less represented voices are heard and that the process does not disproportionately reflect the perspectives of a select few. In the context of our own research, the participants were familiar with focus group settings and often knew one another, which may have influenced the dynamics and outcomes. Alternative group compositions could yield different insights.
If AI can be adapted to incorporate a balance between positive perspectives and realistic assessments of challenges, while addressing these methodological considerations, it may hold significant potential a tool for housing studies and wider public policy research.
This article was originally published on the CaCHE website